Generative AI in Fraud Prevention: Enhancing Data Engineering Workflows to Combat Emerging Threats
Introduction
Fraud has struck financial institutions, healthcare, e-commerce, and telecommunications in today's ever-changing digital ecosystems. In the face of fraudsters' ever-changing methods, organizations that value consumer data, assets, and security prioritize fraud protection. Fraud can generate huge losses in banking, but it can also damage confidence and compromise critical data in healthcare and e-commerce. Modern fraud protection relies on data engineering to collect, transform, and analyze large amounts of behavioural and transactional data. Data engineers build and manage pipelines that use anomaly detection and prediction models to detect fraud in real time. These procedures must be efficient and accurate due to the extent and complexity of digital fraud. Generative AI (GenAI) enhances fraud protection by adding advanced capabilities to data pipelines. Synthetic data, fraud simulations, and pattern recognition allow GenAI to predict issues before they occur. This enables organizations to stay ahead of fraudsters by enhancing detection capabilities and strengthening defences in an ever-evolving threat landscape.
Emerging Fraud Threats in the Digital Era
Fraudsters are adapting to digital transactions and online interactions. Synthetic identity fraud, in which attackers blend real and fake victim information to create new identities and avoid detection, is a major concern. The financial sector is especially prone to this type of fraud because synthetic identities can easily be used to open accounts or apply for loans. Insider fraud, where employees use business technology to perpetrate fraud and evade security protocols, is a growing issue. Another developing issue is real-time payment fraud, where fraudsters transfer stolen money before detection systems can react. Traditional attack detection and prevention systems that use static algorithms and rules may not be able to keep up with cybercriminals' shifting techniques. Scalability is another issue since quickly detecting fraud requires processing large amounts of data from modern digital environments. Due to their simplicity, these algorithms often miss synthetic identity fabrication and other frauds that mix real and phone data. Adaptability is another important problem; new fraud methods may render old models ineffective, leaving businesses vulnerable. System adaptability, scalability, and speed to fraud strategies are needed to combat new threats.
GenAI Enhances Fraud Prevention
Enhanced pattern recognition, data synthesis, and dynamic fraud scenario simulation by generative AI (GenAI) increase fraud prevention efforts.
Pattern Recognition and Anomaly Detection
GenAI finds trends and outliers in massive datasets, many frauds evolve predictably. Traditional fraud detection algorithms may miss these changes due to past data and criteria. GenAI does well discovering fraud anomalies and creating new patterns from old data. GenAI is capable of identifying minor fraud indicators in behavioural data, such as anomalous transaction rates or user interactions, that are overlooked by conventional systems.
Data Synthesis for Fraud Detection
The lack of fraudulent transactions compared to genuine transactions makes training fraud detection models difficult. Fraudulent acts are rare, making it difficult to build solid models with few examples. GenAI generates synthetic data to replicate genuine and fraudulent financial transactions. More balanced datasets improve machine learning model accuracy and fraud detection. Synthetic data helps models learn from fraud scenarios to improve generalisability and detect complex fraud schemes in real life.
Dynamic Fraud Scenario Simulation
GenAI simulates evolving fraud situations to train and evaluate fraud detection systems. GenAI simulations of real-life fraud scenarios with different complexity and approaches keep fraud detection systems adaptable and responsive. Dynamic testing makes systems more adaptable to evolving fraud strategies. GenAI improves fraud detection systems' accuracy, adaptability, and efficiency when combined. This helps companies stay up with fraud techniques' rising sophistication.
Integration of GenAI into Data Engineering Workflows
GenAI-powered data engineering procedures boost fraud detection by revolutionizing data collection, preprocessing, analysis, feature engineering, and scalability.
Data Collection and Preprocessing
GenAI accelerates early data engineering by automating purification, normalization, and transformation. To discover and rectify errors or inconsistencies during traditional data preparation may require manual work. Automating this procedure saves time and ensures accurate, consistent, and reliable datasets for fraud detection algorithms.
Real-time Data Analysis
For fraud detection, real-time data analysis is needed. GenAI analyzes user and transaction data in real time, enabling companies to respond rapidly to suspicious activity. Since GenAI examines data streams in real time, it can detect anomalies and fraud immediately. This agility provides an advantage over batch processing and longer reaction times, and it's essential for preventing risks.
Improving Feature Engineering
Fraud detection methods need feature engineering to improve predictions. GenAI generates complicated features from raw data to increase model performance. It identifies data correlations and linkages that are overlooked by conventional methods. GenAI may combine attributes to detect fraud-related user behaviour trends. This expanded feature set helps fraud detection and predictive modelling.
Scalability and Efficiency in Big Data Pipelines
Transactional data grows exponentially as firms expand. GenAI efficiently optimizes large data pipelines and scalable easily. GenAI employs distributed computing resources to process big data sets quickly, accelerating fraud detection. Its scalability allows businesses to maintain performance during huge transaction volumes, enabling accurate and efficient monitoring and detection. GenAI improves data quality and processing speed in data engineering workflows, helping organizations fight fraud in a changing landscape.
Case Studies and Practical Applications
GenAI has increased real-time fraud detection and offered firms cutting-edge tools to handle new and shifting risks in several areas.
Case Study 1: Banking Sector
GenAI is being used by banks to detect real-time credit card and identity theft fraud. GenAI systems at one large financial institution monitor consumer spending in real time to detect and act on suspicious behaviour. The bank can identify fraudulent transactions via synthetic data and real-time fraud detection model training. This proactive technique helps the bank reduce false positives and speed up fraud response times. Consumer confidence rises as bank fraud losses drop.
Case Study 2: E-commerce
GenAI has also enhanced e-commerce fraud detection. GenAI monitors online customer accounts and transactions for fraud for a multinational company. To detect account takeovers and fraudulent transactions, the software evaluates transaction frequency, device information, and browser history. GenAI's data analysis enables the shop to develop a flexible fraud detection system that adapts to new methods. Reduced false fraud warnings have enhanced consumer purchasing experiences and reduced chargebacks.
Ethical Considerations and Challenges
As organizations use GenAI to avoid fraud, ethical issues must be addressed. Balance fraud detection and personal data security is important. AI managing massive sensitive data presents privacy and security concerns. For client data protection and AI fraud detection, organizations need stringent data governance regulations. Legal, compliance, and customer trust require this balance. GenAI model bias is another issue. Biased training data may lead to erroneous fraud detection by AI systems. Biased tactics that target or fail to target demographic groups could hurt persons and fraud detection. Use alternative datasets and frequent audits to eliminate bias and assure model accuracy. GDPR applies to AI-powered fraud detection; Companies should verify their AI policies meet data use, openness, and responsibility criteria. To prevent fraud with GenAI while protecting individual rights and following rules, companies must consider these ethical challenges.
Conclusion: The Future of Fraud Prevention with GenAI
GenAI improves fraud detection data engineering by boosting pattern discovery, real-time analysis, and synthetic data for robust model training. Its talents help organizations adapt swiftly to new fraud methods, boosting security against more complex threats. GenAI can help prevent fraud by utilizing advanced techniques and strategies to improve detection and respond effectively to emerging threats. Businesses should explore incorporating GenAI into their anti-fraud strategies for a more robust and responsive approach. This technology may help companies protect data, create consumer trust, and adapt to the shifting digital economy. Preventing fraud and protecting assets requires aggressive GenAI implementation.
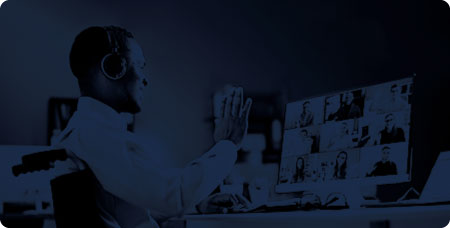