Advanced Fraud Prevention Insights with Machine Learning Experts
If you’re familiar with modern fraud mitigation practices, you’ve likely heard of machine learning. As the advantages of utilizing the vast computational power of machines becomes more pronounced, machine learning is quickly becoming an indispensable tool in a fraud mitigation specialist’s toolbelt.
The fundamentals of machine learning are simple enough; it refers to using computers guided by algorithms to sort through vast quantities of transactional data to detect red flags that a single human, or team of humans, might miss. It’s important to understand beyond just the basics to implement a solution tailored to your unique needs though, and grasping how this field has evolved in recent years should inform your organization’s fraud prevention strategy.
To help contextualize these changes, we spoke with Katherine Wood and Nick Haynes, data scientists from Signifyd, a fraud prevention company that specializes in machine learning and fraud prevention. For additional insights and more education on this important topic, register for the Fundamentals of Machine Learning, an eLearning course explaining the basics and beyond developed by the MRC and Signifyd.
Model Behavior
Before discussing recent developments in the field, it may be useful to examine how machine learning models work to fight fraud.
“Machine learning models can identify red flags in eCommerce orders by ingesting thousands of signals produced by a set of features involved in a transaction,” says Nick Haynes. “Specifically, a model considers identity features, device features, behavior features and history features. The more strongly the patterns in these features match patterns that have resulted in fraudulent orders in the past, the more suspicious the model will be that the order is fraudulent.”
In other words, machines excel at identifying red flags using a wide variety of indicators. Consider the following potential flags that could be important indicators of fraud that a human might not have the bandwidth to examine on a large scale, but a machine could parse quickly:
- Identity Features are anchored by the IP address. What country is it located in? How close is it to the billing address?
- Device ID: Has this device been used in other transactions? What was the outcome?
- Behavior: Does the user navigate directly to a product or do they browse, read reviews, review the merchant’s return policy? What time of day is the purchase made?
- History: How old is the account or email address being used? Has the model seen this shopper before? Has it seen this delivery address?
The above are just a few valuable indicators among many, but It’s important to contextualize these points by looking at the wider picture, says Katherine Wood.
“No one feature tells the whole story, but taken together, patterns emerge. And while many people focus on how those patterns can identify fraudulent orders, even more important is the fact that those patterns can identify legitimate orders that lead to conversions and revenue.”
Machines excel at sorting massive amounts of data and flagging patterns in a way the human brain cannot.
“This ability to automatically extract even complex patterns from data is what allows machine learning models to perform the vast array of tasks they are capable of and to achieve very high performance in many cases,” continues Katherine. “All a model needs to know is how to learn from data, and from there, models can teach themselves how to classify credit card transactions, identify cancerous tumors, or even recommend TV shows.”
How Machine Learning Has Changed
Machine learning is now widely used, but as with any technology, it has evolved quickly. Staying current with that evolution is important for anyone considering implementing a machine learning solution.
According to Nick Haynes, the biggest developments in the field aren’t necessarily in the evolution of machines themselves, but in the power of the tools developing and training the models machines use to sort transactions.
“While academic and industry research groups have continued to push the cutting edge of machine learning forward at an amazing pace in recent years, many of the advances that are making the biggest difference to merchants and fraud prevention professionals have been in tools that make machine learning models easier to train, deploy and manage in a production setting,” says Nick. “The actual model itself is typically only a very small part of the entire machine learning system, and without being able to reliably perform tasks like transforming raw data into a form that can be used by the model, serving predictions in real-time and monitoring the quality of predictions, the best model in the world can't be integrated with other business systems.”
This is an important point, and clearly demonstrates why machines need guidance and can’t operate in a vacuum. The value of providing the right data and guiding the model properly can’t be overstated.
The Human Touch
Though machine learning has come a long way, it’s not yet a silver bullet that eliminates all fraud without the need for human intervention. An effective machine learning strategy involves leveraging the strengths of human insight and contextualization paired with the raw data processing power of a well-trained machine model for ideal results.
“Humans extract very different information from data than machines do,” says Nick. “A human can look at, say, five related transactions, see connections within and between them, and might end up discovering a much larger trend just by observing that small sample. Machines struggle to leverage minuscule amounts of data in this way, but humans can learn from as little as a single example.”
That’s why the ideal machine learning solution is a team of knowledgeable data scientists paired with a model that’s proven to work.
“The best systems rely on a human-plus-machine model,” Nick continues. “A successful human-in-the-loop machine learning system allows each party to focus on what they do best. The model can reduce the amount of tedious work that the domain experts need to perform and allow them to focus on the
most difficult cases where their expertise is the most valuable. In turn, when domain experts identify gaps in the model's performance, they can use this information to improve the model's performance.”
Nick then went on to outline a real-world example, where the context and bigger picture thinking a human can provide becomes invaluable to constructing a cohesive fraud-fighting narrative.
“Humans also bring all their knowledge about the world to bear when looking at data and can use that context when investigating patterns,” he said. “Is this spike in fraud associated with college dorms due to students, or is it fraudsters shipping items to dorms emptied by the pandemic to discreetly retrieve them when no one is around? Did this shopper with a long record of legitimate purchases suddenly start making fraudulent ones because their account was compromised, or are they a victim of a romance scam? These are the kinds of nuanced distinctions that a human can make by investigating transactions deeply, and those findings can be fed back to the system to improve the automated decision-making.”
Education is Key
Payments and fraud prevention continue to evolve at an unprecedented rate, and so do the technologies that power these industries.
Though it can be overwhelming to keep up with the latest developments, the good news is there are extensive resources available. In addition to third-party solution providers that can help you integrate technologies like machine learning into organizations of any size, there is also a growing library of educational resources available as well, allowing you and your team to get up to speed on all the latest developments and stay current.
For further education specifically around machine learning and how it can be used to further your team’s fraud-fighting efforts, explore the Fundamentals of Machine Learning course created by the experts at Signifyd.
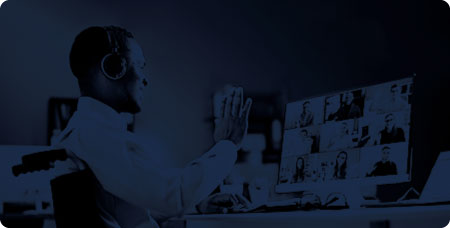