Fraud Networks: Our Answer to Detecting Fraudster Webs
Conventional fraud detection has historically been centered around linear relationships between user indicators. Machine learning techniques in this domain also focus on learning and adapting to patterns arising from single depth connections of datapoints. The weak point of this setup is that we miss out on transitive relationships, which becomes an increasingly interesting factor when fraudsters reuse few overlapping indicators in new fraud attempts.
At Booking.com, we solved this problem by using graph technology to power our payment fraud controls. In this presentation we will show how we developed an in-house technological ecosystem that stores payments transactions in graph format and leveraged state of the art algorithms to compute innovative graph features that enriches our existing fraud controls (ML models and static rules). We will have a look at the different parts of the system: a latency sensitive real time feature computing service, a visualization tool for analysis of networks and a historical feature reconstruction mechanism. Finally, we will have a look at the impact we saw on our fraud controls after incorporating the new features.
We would conclude by explaining the plans to scale this technology in other fraud domains like marketing & rewards, account takeover etc. and exploring Network Representation Learning (a type of deep learning on graph data) to tackle them.
Some content is hidden, to be able to see it login here Login
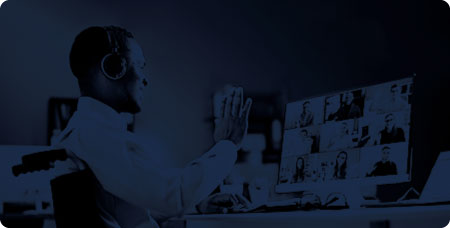