Fake Reviews: A Five-Star Strategy to Prevent Brand Abandonment
Fraud
Attack Types
Machine Learning
Review fraud
Brittany Allen -- Sift
Oct 18, 2020
Presentations
Customer reviews can be an effective way for consumers to learn more about a product or service prior to purchasing. On the other hand, sham reviews can quickly lead to a loss of trust. Here, Sift looks at why product reviews matter and why fake reviews pose a growing problem to online retailers. The focus then turns to content moderation and a five-part solution centered on machine learning to combat phony reviews, with a set of best practices concluding the deck.
Some content is hidden, to be able to see it login here Login
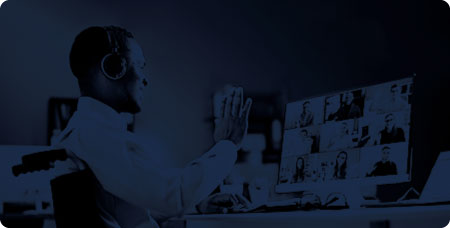
Host a Webinar with the MRC
Help the MRC community stay current on relevant fraud, payments, and law enforcement topics.
Submit a Request
Publish Your Document with the MRC
Feature your case studies, surveys, and whitepapers in the MRC Resource Center.
Submit Your Document