How Can AI and ML Help Reduce False Declines?
By Kevin Siegel, SVP Decision Science & Analytics
Online transactions have become increasingly popular over the years, with more customers opting for the convenience of shopping and paying online. However, as online transactions have increased, so has the risk of fraud.
Many online merchants have implemented fraud prevention systems that use complex algorithms to detect potential fraudulent transactions in order to protect their businesses and customers.
While fraud prevention systems are essential, they can sometimes lead to false declines. This is when legitimate transactions are wrongly identified as fraudulent and then rejected. False declines not only inconvenience customers but also result in lost revenue for businesses.
This article will examine the issue of false declines and explore how artificial intelligence (AI) and machine learning (ML) can help address this problem. We will first define false declines and examine their causes and consequences for both businesses and customers. Next, we’ll provide an overview of how ML fits into this new strategy to reduce false declines using AI.
Additionally, we’ll examine case studies of businesses that have successfully implemented ML in fraud prevention and detection, and the impact of these technologies on false declines. Finally, we will provide recommendations for businesses looking to implement ML strategies in fraud prevention and detection to reduce false declines while enhancing the customer experience.
Understanding False Declines
So, what are false declines? False declines occur when a legitimate transaction is flagged as fraudulent and declined by a merchant's fraud prevention system. Unfortunately, false declines are a result of the imbalance between the need to prevent fraud and the need to accept legitimate transactions. While fraud prevention systems are essential for protecting businesses and customers from fraudulent transactions, false declines can have significant consequences.
There are several causes of false declines, including outdated fraud prevention systems, complex and rapidly changing fraud patterns, and insufficient data analysis. In some cases, fraud prevention systems may be overly cautious, leading to legitimate transactions being incorrectly flagged as fraudulent—definitively, false declines/false positives. Moreover, the increasing sophistication of fraudsters, such as the use of stolen identities and advanced hacking techniques, further complicates fraud prevention efforts, leading to an increased risk of false declines.
The consequences can be severe for both businesses and customers. For businesses, false declines can result in lost revenue and damage to their reputation. For customers, false declines can be frustrating and inconvenient, leading to a negative experience with the merchant. In some cases, false declines can even result in customers taking their business elsewhere, leading to long-term damage to the merchant's reputation and bottom line.
While fraud prevention systems are necessary to protect against fraudulent transactions, it is essential to strike a balance between preventing fraud and accepting legitimate transactions. In the next section, we will explore how ML can help address the issue of false declines.
How AI and ML Can Help With False Declines
At its core, ML is a subset of AI that focuses on the development of algorithms that enable computers to learn from data and improve their performance over time. These technologies have shown promise in reducing false declines by improving fraud prevention systems. On a broader level, AI refers to the development of computer systems that can perform tasks that typically require human intelligence, such as reasoning, learning, and decision-making.
If the goal is to prevent false declines, the path towards achieving that goal should include a wholistic data science approach that includes rules, anomaly detection, AI technologies, fraud filters, limited manual review, and link analysis.
Specifically, ML can help reduce declined transactions for legitimate customers in several ways.
- Machine learning can analyze large amounts of data and identify patterns that may be difficult for humans to detect. By identifying patterns of legitimate transactions, the algorithms that power ML solutions can improve the accuracy of fraud detection systems, reducing false positives and false negatives.
- Data science technologies can adapt to changes in fraud patterns over time. Fraud patterns and techniques used by fraudsters are constantly evolving, making it challenging for traditional fraud prevention systems to keep up. AI solutions can analyze new data and adapt to changes in fraud patterns, making fraud prevention systems more effective in detecting and preventing fraudulent transactions.
- AI and ML can improve the speed and efficiency of fraud prevention systems. Traditional fraud prevention systems may take a long time to analyze data and detect fraudulent transactions, leading to delays and inconveniences for customers. With ML, fraud prevention systems can analyze data in real-time and detect potential fraudulent transactions more quickly, reducing the risk of false declines and improving the customer experience.
AI and ML have the potential to improve fraud prevention systems and reduce the risk of false declines. By analyzing large amounts of data, adapting to changes in fraud patterns, and improving the speed and efficiency of fraud prevention systems, these ever-advancing technologies can enhance the accuracy of fraud detection and prevent legitimate transactions from being declined. Let’s explore some recommendations for businesses looking to implement ML in fraud prevention and detection.
Case Studies
Several businesses have successfully implemented some form of artificial intelligence in their fraud prevention systems to reduce false declines. One such example is PayPal, which uses machine learning to identify fraudulent transactions by employing neural networks to analyze data from billions of transactions and identify patterns of fraudulent activity.
Another example is Mastercard, which has partnered with Vesta to deliver state-of-the-art fraud management solutions in both Latin America and Asia Pacific—two regions rich in emerging eCommerce markets. The partnership includes a deep understanding of machine learning as part of a full suite of fraud prevention tools to improve accuracy and reduce false declines. Mastercard leverages Vesta’s market-leading cyber capabilities to analyze data and identify patterns that may indicate fraudulent activity throughout these regions.
Conclusion
False declines can have severe consequences for businesses and customers, leading to lost revenue, damage to reputation, and negative customer experiences. AI and ML have the potential to improve fraud prevention systems and reduce the risk of false declines by analyzing large amounts of data, adapting to changes in fraud patterns, and improving the speed and efficiency of fraud prevention systems.
Businesses looking to implement a machine learning strategy in fraud prevention and detection should consider several factors, such as the quality and quantity of data, the level of expertise needed to develop and implement artificial intelligence algorithms, and the potential costs and benefits of implementing these systems.
Overall, AI and ML offer promising solutions to the problem of false declines, and businesses that implement these technologies in their fraud prevention systems are likely to see improvements in fraud detection accuracy, reduced risk or false declines, and enhanced customer experiences.
About Vesta
Vesta is a global transaction guarantee company for online purchases, delivering unparalleled approval rates and a frictionless customer experience while eliminating chargebacks and other forms of digital fraud. Differentiated by its sophisticated data science and 25+ years of experience, Vesta brings a deep understanding of some of the world’s most serious threats and challenging markets. Leading brands in ecommerce, travel, financial services, and telco rely on Vesta to provide accurate decisions and 100% guaranteed transaction and revenue protection in real time.
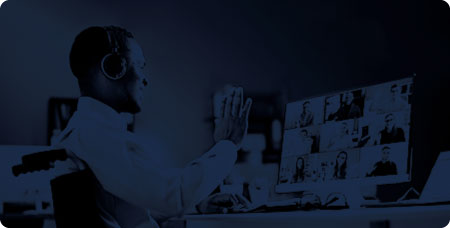